Media bias detection in the news
Unbiased and fair reporting is an integral part of ethical journalism. Yet, political propaganda and one-sided views can be found in the news and can cause distrust in the media. Both accidental and deliberate political bias affect the readers and shape their views. Thus, an important use case for our research is to analyze news and social media text in order to identify problematic, agenda-setting, toxic or misleading information on the Web. This is a shared mission with the Hasso-Plattner-Institut in Potsdam, Germany and Factmata, a UK-based company that fights misinformation on the Web and promotes balanced and fair online content.
Master student project: Hyperpartisan news detection
In our master course Enterprise Data Management (EDM) 2018/2019, we have worked with students on the Semeval 2019 competition dataset with the goal to identify highly politically biased (hyperpartisan) news articles. This competition is organized by Factmata and the research group Webis. In the depicted figure, you can see some initial classification results of our students, which are achieved by using basic Deep Learning techniques, i.e. the Fasttext classifier.
People
Lukas Danke, Lennart Egbers, Michael Harms, Benjamin Schurian (master students)
Konstantina Lazaridou (project advisor, PhD student)
Prof. Alexander Loeser (main supervisor)
Read more
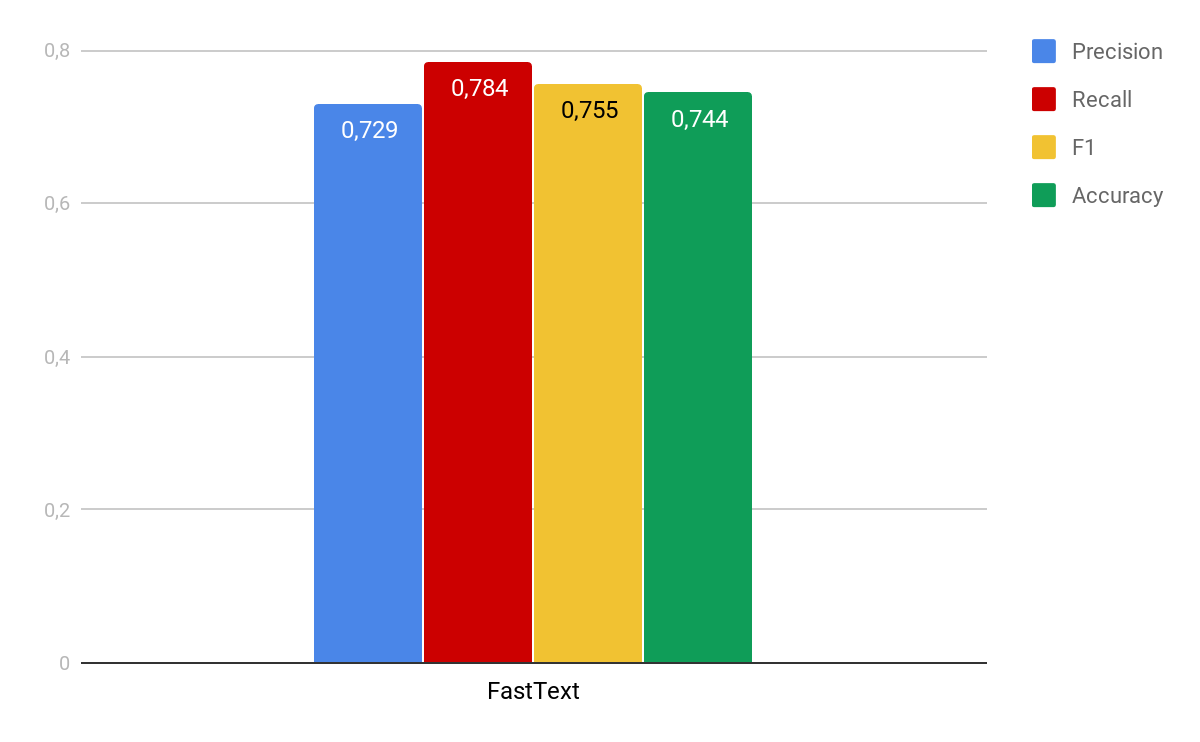
Doctoral research: Ongoing study for media bias detection leveraging human expertise
We classify political news articles with deep learning techniques, while keeping humans-in-the-loop during the training process of our model. We use news articles annotated by different communities, i.e., domain experts and crowd workers, and we also consider automatic article labels inferred by the newspapers' ideologies. Using deep neural networks and self supervision we achieve very promising classification results.
Konstantina Lazaridou, Alexander Löser, Maria Mestre, Felix Naumann : Discovering Biased News Articles Leveraging Multiple Human Annotations. LREC 2020
People
Konstantina Lazaridou (PhD student)
Prof. Alexander Loeser, Prof. Felix Naumann (advisors)
Bachelor thesis: Browser plugin development for online hate speech and media bias
We develop a plugin for Google Chrome that given the text of a webpage or a highlighted text from the user outputs two scores: the levels of hateful language and political bias in the input text. The motivation behind this tool is to assist the news readers and social network users and protect them from misleading or toxic content. Another use case of our tool is that it can provide useful training data for machine learning tasks. The models for hate speech and bias detection are neural networks that we have developed in our research team for these tasks.
More information about the models and the link to our plugin will be published soon here.
People
Sebastian Voigt (Bachelor student)
Betty van Aken, Konstantina Lazaridou (technical advisors, PhD students)
Prof. Alexander Loeser (thesis supervisor)